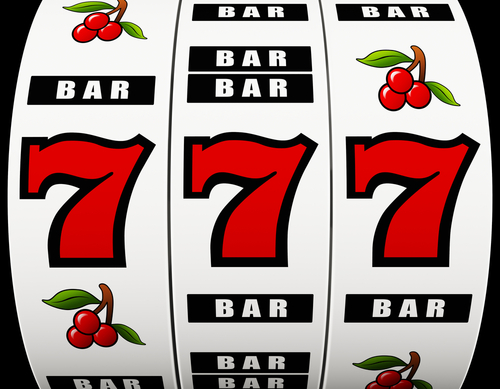
Two milestones happened this week in genomics: the 1000-genomes project wrapped up, and pacbio announced a new machine. The 1kg papers are setting the new minimum for high-impact “large” genomics studies: cohorts on the order of 1000 genomes (funded by a dedicated R01 or consortium). Any large scale switch from Illumina would have to be able to sequence this many samples.
Genomics studies using pacbio sequencing will likely target structural variation since Amplified and Cycled Sequencing (ACS*, Illumina for example) is just fine for SNVs. My current methods for structural detection need more than 10x coverage, though they were hastily written and I will try to make coverage requirements go down with better methods.
For the time being, for a 30x genome on sequel, many rough estimates are placing a pacbio based human genome between $10k-20k. Before you spend any time debating that, remember this is a moving target with many variables for actual cost at play. For example, our cost center charges around $800/SMRTCell, and this includes overhead of labor and cost amortization of the instrument. The lower instrument cost and fixed labor cost means a 3x increase in SMRTCell doesn't translate to a 3x increase in total run cost.
For disorders where there is missing heritability, we may see some <100 genome pilot studies launched - consider the Gilissen et al paper http://www.ncbi.nlm.nih.gov/pubmed/24896178 which demonstrated an increase in diagnostic yield moving from WES to WGS. Still, much of the variation detected in this paper is coding regions missed by exome captures but picked up in WGS. The 3 percent or so of genes missed by ACS reads isn't likely to explain the remainder of missing heritability, and so we'll be looking for new variation in noncoding sequences.
Large scale WES studies were pretty much guaranteed to have significant results -- either the causal mutations in Mendelian disorders or enough likely causal genes in simplex cohorts to improve our understanding of a disease. The noncoding space is a bit more unpredictable. There are plenty of examples of disrupted enhancers. The SHH mutation in polydactyly comes to mind, though this is a point mutation. If more examples of small SVs taking out functional regulatory elements are found, it can certainly help increase our understanding of regulatory architecture. Also, consider that the FTD-ALS locus was not detected until several years ago after a massive dedicated effort from multiple groups. The initial human SMS studies are finding lots of tandem repeat variation, perhaps there are other disorders lurking in our genomes?
The short answer is that an SMS based study of human disease is almost guaranteed. I apologize for the trite ending, but in the long run, the question will be what will human genetics mean for SEQUEL?
*This is my acronym for NGS/HTS/short-reads, and I'd love for it to catch on.